In the annals of technological history, there are moments that epitomize seismic shifts—the point when electricity transitioned from a novelty to a household necessity or when the Internet evolved from a luxury to the backbone of global connectivity. Today, we stand at such an inflection point, courtesy of Generative Artificial Intelligence (GenAI).
Transformation from data science to domain wisdom
At the heart of this revolution is a significant shift from the complexities of data science to the value of domain expertise. Foundational models, meticulously pre-trained, have already done the heavy lifting, thereby changing the locus of focus. Now, it’s not about how well-versed you are in machine learning algorithms but about how richly you understand your industry, your customer, and your unique market differentiators. Whether you’re a burgeoning startup or a traditional enterprise that has previously lagged in technological agility, GenAI stands ready to amplify your innate business wisdom.
Orchestrating scalability and value in GenAI adoption
Like the gilded pages of a classic novel, GenAI’s true worth lies not just in its intrinsic promise but in how adeptly you turn its pages to suit your enterprise narrative. To that end, it’s imperative to strategize for scalable, responsible, and value-driven implementation.
Consider the multitude of AI models that an expansive organization might currently be grappling with—possibly a convoluted arsenal numbering in the hundreds. GenAI ushers in the kind of simplification that can only be termed transformative. With pre-trained foundation models, you’re not just alleviating the need to spawn a new model for each fresh use case, you’re essentially streamlining the entire architectural framework of your data-driven strategies.
7 Key architectural considerations for GenAI
As the captains steering the enterprise ships, business leaders are entrusted with more than just the ‘why’ of GenAI; they must also grapple with the ‘how.’ To navigate these waters adeptly, we recommend an incisive self-inquiry through seven critical aspects, a heuristic roadmap for Generative AI readiness.
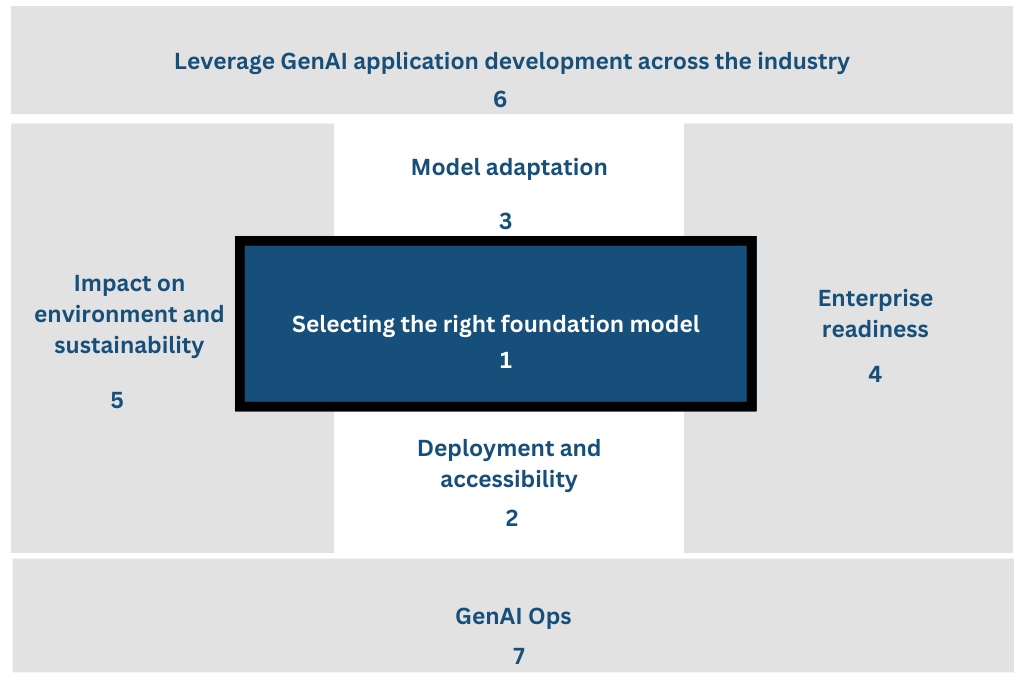
Source & Copyright: Accenture
-
Selecting the foundation model
-
Making the foundation model accessible
-
Adapting these models for data consumption
-
Considering the readiness of enterprise
-
Environmental impact
-
Leveraging Gen AI App development in industry
-
Operating Gen AI at scale
In the ever-evolving realm of artificial intelligence, there stands a beacon of transformation—Generative AI (GenAI). This transformative shift promises not just incremental change but an entirely new paradigm. However, with innovation comes the inevitable question: How do enterprises adapt and adopt? Allow me to shed light on seven crucial considerations every forward-thinking leader must ponder in the GenAI age.
Ideal foundation model
The marketplace is bustling with Generative AI models, each boasting unique merits. Be it pure-play vanguards like OpenAI, the treasure trove of open-source models offered by platforms like Hugging Face, or cloud titans venturing into full-stack services. While the increasing accessibility and affordability of models, democratizes GenAI, the challenge is selecting a model tailored to your organizational aspirations.
Accessibility
Atish demystifies the GenAI accessibility conundrum by presenting two avenues—asserting “full control” by leveraging your own infrastructures, or prioritizing agility through managed cloud services. Both paths have distinct advantages. Yet, embarking on the ‘full control’ route requires meticulous planning, from infrastructure optimization with technologies like Nvidia’s GPUs to addressing next-gen computation demands via disruptors.
Tailoring models to enterprise data
The secret source to GenAI’s efficacy often lies in the harmonious melding of foundational models with proprietary enterprise data. Think of it as the “buy, build, or boost” strategy. Whether you’re integrating pre-trained models with in-context learning, enhancing them with your data, or crafting bespoke models, the essence is in leveraging an agile data foundation.
Assessing enterprise readiness
While technical proficiency is paramount, the true test lies in embedding GenAI within the fabric of enterprise norms. The integrity of these models hinges on addressing the trifecta of security, reliability, and responsible AI. As businesses dance with Generative AI, refining AI governance standards and formulating customized controls becomes indispensable.
Decoding environmental equation
The power of Generative AI is not devoid of environmental considerations. Adapting and fine-tuning these behemoths can escalate energy consumption. This not only questions the sustainability quotient but also the organization’s ecological footprint. Tools like Accenture’s Green Cloud Advisor serve as guiding lights in this journey.
GenAI application development
With a foundational model at your behest, the stage shifts to framing robust frameworks to turbocharge application development. Harnessing the prowess of vector databases, domain knowledge graphs, and prompt engineering can yield a repository of dynamic prompts and templates, setting the stage for GenAI excellence.
Operating GenAI at scale
As enterprises traverse the GenAI landscape, the MLOps paradigm—traditionally used for machine learning—must evolve, making room for AI nuances. This metamorphosis is not just about retrofitting processes but sculpting an operations architecture that’s agile and responsive.
Harmonizing architecture to unlock the potential of GenAI
Navigating the intricate corridors of architectural decisions sets the stage for an enterprise to unleash the full power of Generative AI (GenAI). By addressing architectural complexities, organizations sculpt an agile and robust framework that not only maximizes efficiency but also paves the way for seamless GenAI adoption throughout the enterprise. Yet, this is merely one dimension of a multi-faceted success equation.
We must acknowledge an irrefutable truth: Technological excellence alone is insufficient to realize the transformative potential of GenAI. The human element—spanning both the technically proficient and those yet to be acquainted with the complexities of AI—holds equal, if not greater, significance.
The velocity of change in generative AI and its ecosystem is such that rigid plans or monolithic architectures may soon become obsolete. Thus, both organizational and IT agility are prerequisites for fully harnessing the transformative jolt brings to the AI landscape.
In summary, the holistic approach to succeeding in the Generative AI paradigm necessitates a symbiotic relationship between advanced architectural frameworks and an agile, skilled, and adaptable human capital. As the ecosystem of capabilities around foundation models continually evolves, staying both adaptive and responsive becomes the cornerstone for capturing the revolutionary value that GenAI promises to deliver.