Have your data questions been answered yet?
It is less about data and more about insight. Focus on the things which matter.
The potential of Big Data is in its ability to solve business problems and provide new business opportunities. So to get the most from your Big Data investments, focus on the questions you’d love to answer for your business. This simple shift can transform your perspective, changing big data from a technological problem to a business solution.
The facts you need are likely buried within a jumble of legacy systems, hidden in plain sight. Big Data can uncover those facts, but typical analytics projects can turn into expensive, time-consuming technology efforts in search of problems to solve.
Our lean learning approach measures the value you gain at each step, in small iterations. Use the results to improve the process or change course to a more fruitful direction.
Big Data got its start in the late 1990s to early 2000s when the largest Internet companies were forced to invent new ways to manage data of unprecedented volumes. Today, most people think of Hadoop or NoSQL databases when they think of Big Data.
However, the original core components of Hadoop, HDFS (Hadoop Distributed File System – for storage), MapReduce (the compute engine), and the resource manager now called YARN (Yet Another Resource Negotiator) are rooted in the batch-mode or offline processing commonplace ten to twenty years ago, where data is captured to storage and then processed periodically with batch jobs. Most search engines worked this way in the beginning. The data gathered by web crawlers was periodically processed into updated search results.
Client Stories
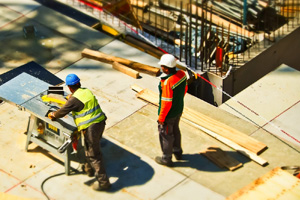
Labour Analytics Dashboard,
Ministry of Labour & Employment, Government of India
We have built a POC system which would accurately project worker force availability and other labour parameters for the next 30 years.
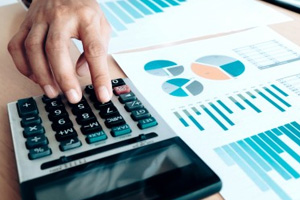
Quick Financials, Proactive Tech Systems
This cloud based SaaS system analyzes your company’s financial statements and gives you a better idea of the financial health of your business.
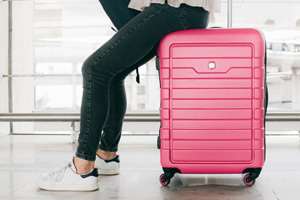
USA Travels
Used our solution to protect and increase revenue by using data insights to understand fluctuations in sales volumes and adjust price strategy (Dynamic pricing policy) accordingly.
At the other end of the processing spectrum is real-time event processing, where individual events are processed as soon as they arrive with tight time constraints, often microseconds to milliseconds. The guidance systems for rockets are an example, where behavior of the rocket is constantly measured and adjustments must be made very quickly. Between these two extremes are more general stream processing models with less stringent responsiveness guarantees. A popular example is the mini-batch model, where data is captured in short time intervals and then processed as small batches, usually within time frames of seconds to minutes. The importance of streaming has grown in recent years, even for data that doesn’t strictly require it, because it’s a competitive advantage to reduce the time gap between data arrival and information extraction.
For example, if you hear of a breaking news story and search Google and Bing for information, you want the search results to show the latest updates on news websites. So, batch-mode updates to search engines are no longer acceptable, but a delay of a few seconds to minutes is fine. Stream processing is also being used for these tasks:
- Updating machine learning models as new information arrives.
- Detecting anomalies, faults, performance problems, etc. and taking timely action.
- Aggregating and processing data on arrival for downstream storage and analytics.
We provide a range of new systems and approaches, which balance various trade offs to deliver timely, cost-efficient data processing, as well as higher developer productivity.